引用本文: |
-
师德强,吴光,李检秀,龙思宇,黄艳燕,黄纪民,王何健,谢能中,严少敏.α-淀粉酶Amy7C及其突变体最适pH值的定量预测[J].广西科学,2014,21(6):664-670. [点击复制]
- SHI De-qiang,WU Guang,LI Jian-xiu,LONG Si-yu,HUANG Yan-yan,HUANG Ji-min,WANG He-jian,XIE Neng-zhong,YAN Shao-min.Quantitative Predicting pH Optimum of α-Amylase Amy7C and Its Mutants[J].Guangxi Sciences,2014,21(6):664-670. [点击复制]
|
|
|
|
本文已被:浏览 440次 下载 436次 |
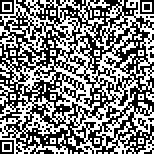 码上扫一扫! |
α-淀粉酶Amy7C及其突变体最适pH值的定量预测 |
师德强, 吴光, 李检秀, 龙思宇, 黄艳燕, 黄纪民, 王何健, 谢能中, 严少敏
|
|
(广西科学院, 非粮生物质酶解国家重点实验室, 国家非粮生物质能源工程技术研究中心, 广西生物质产业化工程院, 广西生物炼制重点实验室, 广西南宁 530007) |
|
摘要: |
[目的]pH值是影响酶催化效率的关键参数,通常需要通过实验方式才能确定生物酶的最适pH值,而该方式要消耗较多的人力、物力和时间。因此,有必要发展一种利用酶的简单结构信息即可预测其最适pH值的方法。[方法]以20-1前馈反向传播的神经网络为模型,完成535种氨基酸属性对α-淀粉酶pH值的拟合。同时,将α-淀粉酶Amy7C及其54个突变体的数据分为2组,用35个酶作为训练组进行拟合,20个酶作为验证组进行检验,并对不同层次及神经元个数的模型进行比较。[结果]109个氨基酸属性可实现20-1神经网络模型收敛,表明这些氨基酸属性可用于预测α-淀粉酶的最适pH值,但是不同氨基酸属性预测pH值的效果差别较大,只有部分指标预测pH值的效果较好。多模型的分析结果显示,不同模型对训练组R值的结果具有显著性差异,而对训练组P值、验证组R值和验证组P值结果无显著性差异。[结论]氨基酸分布概率等属性可用于预测α-淀粉酶的最适pH值。20-1神经网络模型是预测α-淀粉酶最适pH值相对理想的模型。 |
关键词: α-淀粉酶 最适pH值 预测 氨基酸属性 |
DOI:10.13656/j.cnki.gxkx.20141029.001 |
投稿时间:2014-05-10修订日期:2014-06-15 |
基金项目:广西自然科学基金重点项目(2013GXNSFDA019007),广西科技创新能力与条件建设计划项目(桂科能12237022)和广西人才小高地建设专项基金项目资助。 |
|
Quantitative Predicting pH Optimum of α-Amylase Amy7C and Its Mutants |
SHI De-qiang, WU Guang, LI Jian-xiu, LONG Si-yu, HUANG Yan-yan, HUANG Ji-min, WANG He-jian, XIE Neng-zhong, YAN Shao-min
|
(Guangxi Academy of Sciences, State Key Laboratory of Non-food Biomass Enzyme Technology, National Engineering Research Center for Non-food Biorefinery, Biomass Industrialization Engineering Institute, Guangxi Key Laboratory of Biorefinery, Nanning, Guangxi, 530007, China) |
Abstract: |
[Objective] pH is an important parameter in enzymatic reaction, and its determination is often through experimental path, which is generally costly and time-consuming.So, it is necessary to develop methods that can use as simple as possible information to predict pH optimum for enzyme.[Methods] 20-1 feedforward backpropagation neural network was used to screen 535 amino acids properties as predictors to predict the optimal pH of α-amylase Amy7C and its 54 mutant, which were divided as two groups.35 of them served as training group for fitting, and the other 20 were treated as validation.The models for different structures and neuron numbers were also compared.[Results] 109 amino acid properties, which converged during fitting in the 20-1 neural network model, could be used to predict optimal pH.Different amino acid properties presented different predicting effect, and some of them revealed better prediction for optimal pH.The multi-model results showed that there was significant difference between R values in training groups, but there was no significant difference between P values in training groups, as well as R and P values in validation groups.[Conclusion] The distribution probability and some amino acid properties could be used to predict optimal pH of α-amylase, for which 20-1 feedforward backpropagation neural network was the relative ideal model. |
Key words: α-amylase pH optimum prediction amino acid property |
|
|
|
|
|