引用本文: |
-
马子晨,张顺香,刘云朵,王星光,张友强.CCM-MF:基于多维度特征融合的中文文本分类模型[J].广西科学,2023,30(1):35-42. [点击复制]
- MA Zichen,ZHANG Shunxiang,LIU Yunduo,WANG Xingguang,ZHANG Youqiang.CCM-MF:Chinese-text Classification Model Based on Fused Multi-dimensional Features[J].Guangxi Sciences,2023,30(1):35-42. [点击复制]
|
|
本文已被:浏览 255次 下载 355次 |
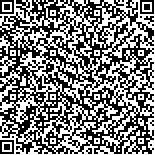 码上扫一扫! |
CCM-MF:基于多维度特征融合的中文文本分类模型 |
马子晨1,2, 张顺香1,2, 刘云朵1,2, 王星光1,2, 张友强1,2
|
|
(1.安徽理工大学计算机科学与工程学院, 安徽淮南 232001;2.合肥综合性国家科学中心人工智能研究院, 安徽合肥 230088) |
|
摘要: |
针对中文文本中不同维度特征所携带的语义信息具有差异性的问题,本文提出一种基于多维度特征融合的中文文本分类模型:CCM-MF (Chinese-text Classification Model Based on Fused Multi-dimensional Features)。该模型融合层次维度和空间维度特征,以提高中文文本分类的准确率。首先,在层次维度上,使用预训练模型ERNIE (Enhanced Representation through Knowledge Integration)获取包含字、词及实体级别特征的词向量;然后,在空间维度上,将包含层次维度特征的词向量分别输入到改进后的深度金字塔卷积神经网络(Deep Pyramid Convolutional Neural Networks,DPCNN)模型及附加注意力机制的双向长短期记忆网络(Attention-Based Bidirectional Long Short-Term Memory Networks,Att-BLSTM)模型中,得到局部语义特征和全局语义特征;最后,将得到的空间维度特征分别作用于Softmax分类器,再对计算结果进行融合并输出分类结果。通过在多个公开数据集上进行实验,较现有主流的文本分类方法,本模型在准确率上有更好的表现,证明了该模型的有效性。 |
关键词: 中文文本分类|多维度|ERNIE|DPCNN|Att-BLSTM |
DOI:10.13656/j.cnki.gxkx.20230308.004 |
|
基金项目:国家自然科学基金面上项目(62076006)和安徽省高校协同创新项目(GXXT-2021-008)资助。 |
|
CCM-MF:Chinese-text Classification Model Based on Fused Multi-dimensional Features |
MA Zichen1,2, ZHANG Shunxiang1,2, LIU Yunduo1,2, WANG Xingguang1,2, ZHANG Youqiang1,2
|
(1.School of Computer Science and Engineering, Anhui University of Science and Technology, Huainan, Anhui, 232001, China;2.Institute of Artificial Intelligence, Hefei Comprehensive National Science Center, Hefei, Anhui, 230088, China) |
Abstract: |
In view of the difference of semantic information carried by different dimensional features in Chinese text,a Chinese-text Classification Model based on Fused Multi-dimensional Features (CCM-MF) was proposed.The model combines hierarchical dimension and spatial dimension features to improve the accuracy of Chinese text classification.Firstly,on the hierarchical dimension,the Enhanced Representation through Knowledge Integration (ERNIE) pre-training model is used to obtain word vectors containing features of character,word,and entity levels.Then,on the spatial dimension,the word vectors containing hierarchical dimension features are input into the improved Deep Pyramid Convolutional Neural Networks (DPCNN) model and Attention-Based Bidirectional Long Short-Term Memory Networks (Att-BLSTM) model to obtain local and global semantic features,respectively.Finally,the obtained spatial dimension features are applied to the Softmax classifier,and then the calculation results are fused and the classification results are output. Through experiments on multiple public data sets,this model has better performance in accuracy than the existing mainstream text classification methods,which proves the effectiveness of the model. |
Key words: Chinese text categorization|multiple dimensions|ERNIE|DPCNN|Att-BLSTM |