引用本文: |
-
谢金财,于千城,王之赐,胡智勇,于绪龙,王澳强.基于VMD-GRAU的短期风电功率预测[J].广西科学,2024,31(4):773-780,796. [点击复制]
- XIE Jincai,YU Qiancheng,WANG Zhici,HU Zhiyong,YU Xulong,WANG Aoqiang.Short-term Wind Power Forecasting Based on VMD-GRAU[J].Guangxi Sciences,2024,31(4):773-780,796. [点击复制]
|
|
|
|
本文已被:浏览 148次 下载 137次 |
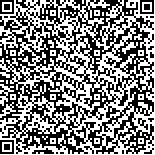 码上扫一扫! |
基于VMD-GRAU的短期风电功率预测 |
谢金财1,2, 于千城1,2, 王之赐1,2, 胡智勇1,2, 于绪龙1,2, 王澳强1,2
|
|
(1.北方民族大学计算机科学与工程学院, 宁夏银川 750021;2.北方民族大学图形图像国家民委重点实验室, 宁夏银川 750021) |
|
摘要: |
针对现有的风电功率短期预测模型难以准确捕捉风电历史监测数据与风电功率之间的非线性映射关系,以及难以适应风速环境变化导致预测效果差的问题,本研究基于变分模态分解(Variational Mode Decomposition,VMD)和门控循环单元(Gated Recurrent Unit,GRU)设计了风电功率预测模型VMD-GRAU。首先对风电数据进行变分模态分解,避免模态混合和假峰值的问题,然后提出门控循环注意力(Gated Recurrent Attention Unit,GRAU)模型,设计注意门来增强GRU对重要序列特征的捕捉能力和稳健性,设计误差修正模块来降低风电功率预测的随机性和波动性影响,最后在损失函数中添加稀疏正则化项来防止模型过拟合。实验结果表明所提出的VMD-GRAU模型在平均绝对误差90(MAE)、均方根误差(RMSE)和拟合优度(R2)方面分别达到0.022、0.016和0.995,相比传统模型GRU和Transformer,本研究提出模型预测的平均绝对误差提升了26%-31%,在不同环境下依然具有较好的泛化性。 |
关键词: 风电功率预测 变分模态分解 注意力机制 正则化 |
DOI:10.13656/j.cnki.gxkx.20241206.016 |
投稿时间:2023-07-19修订日期:2023-09-12 |
基金项目:宁夏重点研发计划(引才专项)项目(2022YCZX0013),宁夏重点研发计划(重点)项目(2023BDE02001),北方民族大学2022年校级科研平台项目“数字化农业赋能宁夏乡村振兴创新团队”(2022PT_S10)和银川市校企联合创新项目(2022XQZD009)资助。 |
|
Short-term Wind Power Forecasting Based on VMD-GRAU |
XIE Jincai1,2, YU Qiancheng1,2, WANG Zhici1,2, HU Zhiyong1,2, YU Xulong1,2, WANG Aoqiang1,2
|
(1.School of Computer Science and Engineering, North Minzu University, Yinchuan, Ningxia, 750021, China;2.Laboratory of Graphics and Images of the State Ethnic Affairs Commission, North Minzu University, Yinchuan, Ningxia, 750021, China) |
Abstract: |
The existing short-term wind power prediction models are difficult to accurately capture the nonlinear mapping relationship between wind power historical monitoring data and wind power and adapt to the environmental changes in wind speed,which lead to poor prediction results.In view of these problems,a wind power prediction model was designed based on Variational Mode Decomposition (VMD) and Recurrent Neural Network (RNN).The model first performed VMD of the wind power data to avoid the problems of mode mixing and false peaks,and proposed the Gated Recurrent Attention Unit (GRAU) model,which designed an attention gate to enhance the ability of RNN to capture the important sequential features and robustness.An error correction module was designed to reduce the effects of stochasticity and volatility in wind power prediction,and finally a sparse regularization term was added in the loss function to prevent the model from being overfitted.The experimental results showed that the proposed VMD-GRAU model achieved the average values of 0.022,0.016,and 0.995 in terms of Mean Absolute Error (MAE),Root Mean Square Error (RMSE),and R2 score,respectively.The model proposed in this study improved the MAE by 26%-31% compared with GRU and Transformer models and had good generalization performance in different environments. |
Key words: wind power prediction variational mode decomposition attention mechanism regularization |
|
|
|
|
|